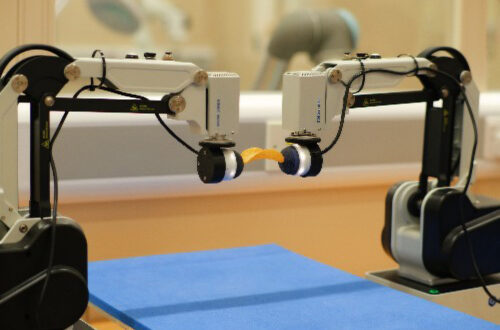
In robotics, achieving human-like dexterity has always been a coveted milestone. Human hands’ intricate movements, sensitivity, and adaptability have long been benchmarks, challenging roboticists worldwide. Today, we stand on the cusp of a significant breakthrough.
A groundbreaking bimanual robot with two arms showcases tactile sensitivity that mirrors human-level dexterity.
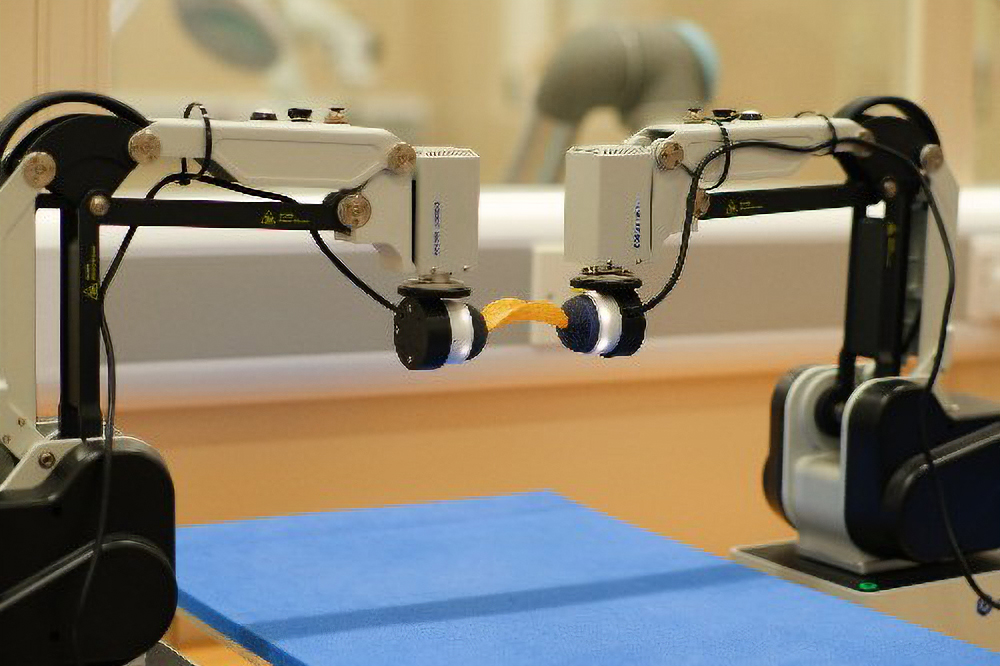
By harnessing the power of artificial intelligence (AI), this robot is redefining what’s possible, blurring the lines between human capabilities and machine precision. The world of robotics is no stranger to innovation. We’ve seen remarkable advancements, from industrial robots that can assemble intricate machinery to humanoid robots that can dance and converse. However, the challenge has always been to replicate human hands’ nuanced movements and tactile sensitivity.
The Bi-Touch System
The robot is based on a Bi-Touch system Developed by researchers at the University of Bristol and based at the Bristol Robotics Laboratory.
The primary objective of the Bi-Touch system is to enable robots to perform manual tasks that were once deemed too intricate for machines.
By leveraging AI, the system can interpret and respond to its environment, making real-time decisions. But what sets it apart is its ability to “sense” through tactile feedback, much like how humans rely on touch to interact with the world around them.
The robot can gauge objects’ texture, temperature, and other physical attributes by integrating tactile sensors, allowing for more nuanced interactions. It’s designed to learn and adapt. As the robot interacts with its environment, it continually refines its actions, ensuring optimal performance. This adaptability is crucial, especially when handling delicate or unfamiliar objects.
The Bi-Touch system is a bridge between the digital and physical worlds. It allows the robot to gather data, process it through AI algorithms, and execute actions with precision and sensitivity. At the core of the robot’s capabilities is its ability to interpret its surroundings. Using AI, the robot can process vast amounts of data in real time, allowing it to make informed decisions. Whether identifying an object’s shape or understanding its material properties, the AI agent acts as the robot’s brain, guiding its actions based on the information it receives.
For the robot to truly mimic human-like dexterity, it must “feel.” This is where tactile and proprioceptive feedback comes into play. Tactile feedback provides the robot with information about the surface and texture of objects. It can discern the difference between a soft sponge and a hard metal, allowing it to adjust its grip strength accordingly. Proprioceptive feedback, on the other hand, gives the robot a sense of its body position and movement. The robot can precisely execute intricate tasks by understanding its orientation and the status of its limbs.
Learning Through Touch
One of the standout features of the bimanual robot is its ability to learn through touch. As it interacts with various objects, the robot refines its actions based on the feedback it receives.
This iterative learning process, powered by Deep Reinforcement Learning, allows the robot to improve its performance over time. It’s a dynamic system where each interaction is a learning opportunity, enabling the robot to adapt to new challenges.
By combining the computational capabilities of AI with the tangible insights from sensory feedback, the robot can navigate its environment with a level of sophistication previously unseen in the world of robotics.
Applications and Implications
The emergence of a robot with near-human dexterity, powered by the Bi-Touch system, opens up many possibilities across various sectors. The potential applications are vast, and the implications for industries and society are profound.
Healthcare and Rehabilitation
One of the most promising areas for the bimanual robot is healthcare. With its delicate touch and precision, the robot can assist in surgical procedures, ensuring accuracy and minimizing human error. Furthermore, the robot can aid patients in physical therapy and rehabilitation, providing consistent and adaptive support.
Manufacturing and Assembly
The bimanual robot can be pivotal in industries where precision is paramount. From assembling intricate electronic components to handling delicate materials, the robot’s tactile sensitivity ensures that tasks are executed with utmost care. This not only enhances production quality but also reduces wastage and errors.
Research and Laboratory Work:
The robot’s ability to handle delicate objects, such as lifting a single Pringle crisp, showcases its potential in research settings. Whether taking fragile specimens or conducting precise experiments, the robot can assist researchers in tasks that demand a gentle touch.
Assistive Technologies:
Beyond industrial applications, the bimanual robot holds promise in assistive technologies. For individuals with mobility challenges, the robot can serve as an extension, aiding in daily tasks and improving the quality of life.
The advent of the bimanual robot is not just about technological advancement; it reflects the evolving relationship between humans and machines. As robots become more integrated into our daily lives, questions about ethics, job displacement, and societal adaptation arise. While the robot offers numerous benefits, navigating these challenges with foresight and responsibility is essential.
Deep Reinforcement Learning in Robotics
Deep Reinforcement Learning (DRL) is one of the pillars behind the bimanual robot’s remarkable capabilities. This advanced AI technique, often associated with teaching machines through trial and error, enables the robot to achieve its human-like dexterity.
Deep Reinforcement Learning is about learning optimal strategies through interaction with an environment. Unlike traditional learning methods, where machines are fed labelled data, DRL allows robots to explore, make decisions, and learn from the outcomes. Each action produces a reward or penalty, guiding the machine towards optimal behaviours over time.
As the robot interacts with objects, it continually refines its grip, movement, and overall approach based on the feedback it receives. Each interaction, whether successful or not, serves as a learning point, allowing the robot to enhance its performance incrementally.
One of the features of DRL is its adaptability. Since the learning process is dynamic, the robot can adjust to new challenges and environments. This adaptability is crucial, especially when handling unfamiliar or delicate objects. The robot doesn’t just rely on pre-programmed instructions; it evolves based on its experiences, ensuring a high degree of flexibility in its actions.
Deep Reinforcement Learning leverages intense neural networks to process and interpret vast amounts of data. These networks, inspired by the human brain’s structure, allow the robot to make sense of its tactile and proprioceptive feedback, guiding its actions precisely.
As research advances, we can expect even more sophisticated robots capable of navigating complex environments and tasks. By learning through interaction, refining its approach through trial and error, and leveraging the power of neural networks, the robot showcases what’s possible when machines can “think” and “feel.”
As we stand on the cusp of this new era, it’s essential to approach the future with both excitement and responsibility. While the bimanual robot offers a glimpse into a world of endless possibilities, it also raises questions about ethics, societal adaptation, and the broader relationship between humans and machines. Navigating these challenges will be crucial as we move forward.
TLDR:
- A groundbreaking bimanual robot demonstrates near-human dexterity, powered by the Bi-Touch system.
- Developed by researchers at the University of Bristol, the robot can interpret and respond to its environment using AI and tactile feedback.
- Deep Reinforcement Learning (DRL) enables the robot to learn through interaction, refining its actions based on feedback.
- Potential applications span across healthcare, manufacturing, research, and assistive technologies.
- The robot’s capabilities highlight the future of robotics, where machines can seamlessly integrate into daily life and adapt to complex tasks.
- Ethical and societal implications arise as robots become more integrated, necessitating responsible navigation of the future.