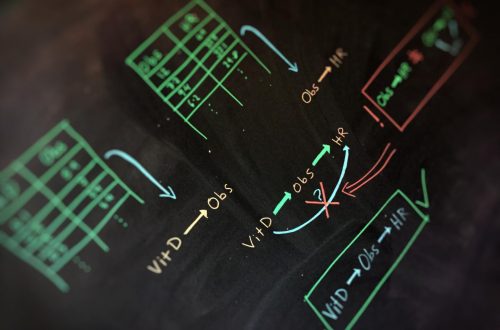
New Artificial Intelligence (AI) tech has been developed that can merge overlapping and incomplete medical datasets to determine which variables are causative.
How artificial intelligence tackles establishing causation from correlation
The technique, developed by Babylon Health, could breathe new life into old or incomplete datasets after proving its worth against data from tumours and protein structures.
The approach is thought to be a the first time computer scientists have been able to demonstrate a useful and reliable way of sorting through large amounts of correlating data to spot when correlation means causation.
The team tested the program using old, overlapping and incomplete datasets and hope it could be used to better understand the results of medical trials that would otherwise be too expensive or even unethical.
The research was a result of exploring new ways to help spot causative variables. The team-based their approach on the idea of entropy. Entropy is a core idea in physics that says everything becomes more disordered and complicated with time. This means that if something is “a cause” it should be less disordered and complex than its effect.
Using this theory the AI can then take a dataset and give each of the variables a complexity rating, it then works backwards and spots which one is the cause.
While this is obviously useful to researchers the AI is potentially a game-changer when it comes to combining datasets. Dr Ciarán Lee, a Senior Research Scientist at Babylon and Honorary Senior Research Associate at UCL, explains that the team were inspired by quantum cryptography.
Quantum cryptography offers a mathematical formula to prove whether someone else is eavesdropping on a conversation.
The team took this algorithm and were able to apply it to datasets in a similar way. Instead of spotting potential eavesdroppers they used it to spot potential causative variable from another dataset instead.
According to Dr Lee “if one dataset shows us that obesity causes heart disease, and another shows vitamin D causes obesity we can use a mathematical formula to prove whether vitamin D causes obesity or not. This is what our AI is doing.”
So far the team have tested the AI on breast cancer and protein-signalling datasets, as well as with artificial datasets that were intentionally created to be very complex.
In all of the sets of data, the AI was able to successfully pinpoint the causative variable.
The algorithm used in the research has now been made available in the team’s paper and on the open-access site arXiv so that scientists across the world can use it to reassess overlapping and incomplete datasets.
The team have published the work at the Association for Advancement of Artificial Intelligence (AAAI) conference in New York.